Hi everyone, I’m Hannah, an up-and-coming data consultant from cohort 50! This is my first blog of (hopefully) many at The Data School, where I hope to answer a question that’s been sitting on my mind since I was in the process of applying – what on earth is data cleaning? If, like me, you’ve been reading many blogs posted on here, you will see the phrase “data cleaning” crop up more and more, with lots of people telling me to do it for the interview without understanding what it truly is.
Chances are, if you’ve been using Tableau to make your initial application to The Data School, you have likely already done some data cleaning. Do you need to exhibit your data cleaning skills to get in to The Data School? Absolutely not (otherwise I wouldn’t be here!). This being said, if you don’t want Tableau (or any analytics software) to kick up a fuss and slow you down, its definitely worth giving your data a clean up first.
So, what is it? Data cleaning is simply putting data in the correct structure for analysis. This means avoiding duplicate data, empty cells, and removing information that’s simply irrelevant to the questions that you want to answer using your data. The key here is that computer systems process data differently to us, so we need to accommodate for this so we can get into creating dashboards!
When you get given a fresh set of data, its difficult to get your head around things, let alone understand if the data is clean or not. As a general guideline, “clean” data will often follow a set of golden rules (as always, these rules may change depending on what you want to find):
- Ensure each field (aka column) contains ONE category or measure
- Each field should also only contain ONE data type (it can be all string, Boolean, numerical, etc. as long as it remains consistent throughout your field)
- Each row should represent one record containing all the values in each data field possible
Let’s have a look at what this actually means:
Dirty data:
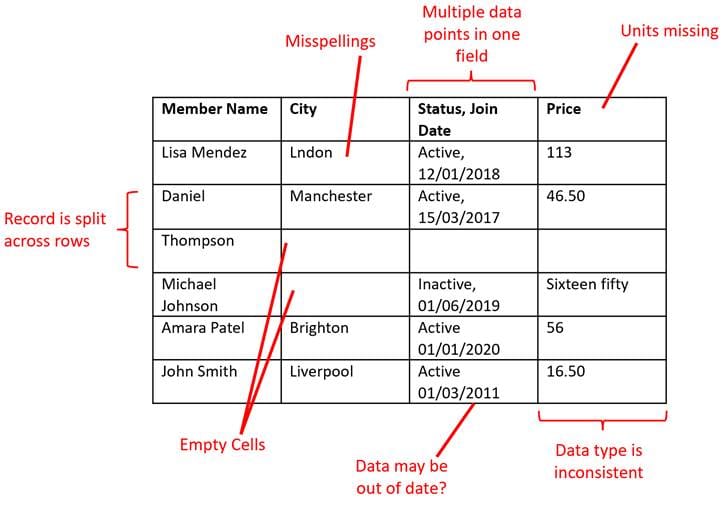
This shows just a few things that can throw a computer off when analysing your data. When working with a client, it's likely worth asking a couple of questions for aspects you're unsure of - such as why nulls are present or if the dataset is out of date.
The same data set all cleaned up:
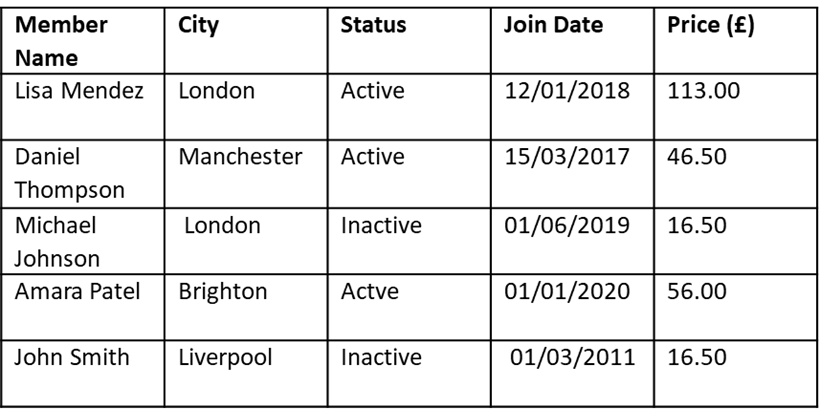
Much better.
When thinking about data cleaning, a useful tip is to ask yourself: what does one row represent? Here each row is showing us a person’s membership status at a specific site and when they joined and how much it cost. I find this particularly useful when a record is split across multiple rows. Data cleaning might sound intimidating at first, but it's really just about making sure your data is easy to work with so you can whip up some gorgeous vizzes!
Whilst this was just a brief overview of data cleaning, I hope it highlights a few key things to look out for when approaching a new set of data. Thanks for reading my first blog here at The Data School — I can’t wait to keep sharing what I learn. Until next time, happy spring cleaning!
H